In this project , we delve into the world of data and databases to analyze various aspects of Telangana’s districts. Our dataset comprises information from multiple tables related to revenue, vehicle sales, and industrial sectors in Telangana. We will conduct SQL queries to extract meaningful insights and provide suitable analysis for each result.
Database Structure
Our database consists of the following tables:
Date: Contains information about months, quarters, and fiscal years.
Districts: Stores district codes and district names.
Stamps: Provides data on document registration and e-stamp challans.
Transport: Contains information about vehicle sales and fuel types.
TS_IPass: Stores data related to industrial investments and employment.
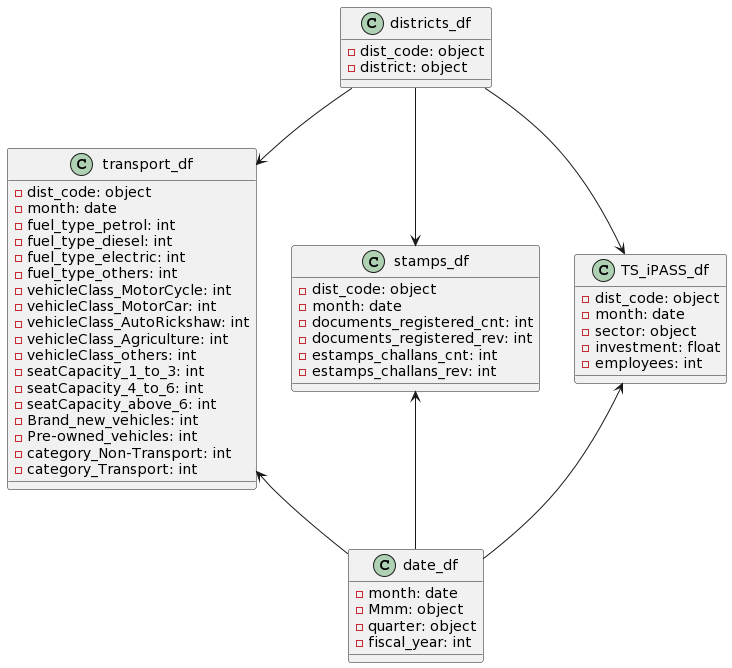
Now, let's dive into the queries and their results:
Analysis
Query 1: Revenue Variation Across Districts
How does the revenue generated from document registration vary across districts in Telangana? List down the top 5 districts that showed the highest document registration revenue growth between FY 2019 and 2022.
SELECT districts.district AS districts, SUM(stamps.documents_registered_rev) AS revenue
FROM districts
JOIN stamps ON districts.dist_code = stamps.dist_code
JOIN date ON stamps.month_ = date.month_
WHERE date.fiscal_year BETWEEN 2019 AND 2022
GROUP BY districts
ORDER BY revenue DESC
LIMIT 5;
Result:
The top 5 districts with the highest document registration revenue growth between FY 2019 and 2022 are:
Rangareddy: $108,198,682,809
Medchal-Malkajgiri: $64,071,382,839
Hyderabad: $38,396,743,983
Sangareddy: $22,024,502,771
Hanumakonda: $7,927,494,100
Analysis:
The results reveal the top 5 districts with the highest document registration revenue growth between fiscal years 2019 and 2022. Rangareddy, Medchal-Malkajgiri, Hyderabad, Sangareddy, and Hanumakonda have emerged as the districts with the most significant increases in revenue.
Rangareddy stands out as the top revenue-generating district, with an impressive document registration revenue growth over the years. This could be attributed to various factors, including a higher volume of property transactions, economic development, or changes in property values in the area.
This information is crucial for understanding the economic landscape in Telangana, and it may guide resource allocation, infrastructure development, and policy decisions within these districts.
Query 2: E-Stamps Revenue vs. Document Registration Revenue
How does the revenue generated from document registration compare to the revenue generated from e-stamp challans across districts? List down the top 5 districts where e-stamps revenue contributes significantly more to the revenue than the documents in FY 2022?
WITH revenue_sums AS (
SELECT
districts.district AS district_name,
SUM(stamps.estamps_challans_rev) AS estamps_revenue,
SUM(stamps.documents_registered_rev) AS documents_revenue
FROM
districts
JOIN
stamps ON districts.dist_code = stamps.dist_code
JOIN
date ON stamps.month_ = date.month_
WHERE
date.fiscal_year = 2022
GROUP BY
district_name
)
SELECT
district_name
FROM
revenue_sums
WHERE
estamps_revenue > documents_revenue
ORDER BY
estamps_revenue - documents_revenue DESC
LIMIT 5;
Result:
The top 5 districts where e-stamps revenue contributes significantly more to the revenue than document registration in FY 2022 are:
Rangareddy
Hyderabad
Khammam
Hanumakonda
Yadadri Bhuvanagiri
Analysis:
This query compares the revenue generated from e-stamp challans and document registration across districts in FY 2022. The results identify the top 5 districts where e-stamps revenue contributes significantly more to the total revenue than document registration.
Rangareddy and Hyderabad are the leading districts in this regard, indicating a strong reliance on e-stamps as a source of revenue. This trend may be influenced by factors such as a shift towards electronic transactions or changes in property registration practices.
Understanding these variations can assist the government in adapting its revenue collection strategies and ensuring a balance between traditional document registration and digital e-stamp processes.
Query 3: E-Stamp Challan and Document Registration Counts
Is there any alteration of e-Stamp challan count and document registration count pattern since the implementation of e-Stamp challan? If so, what suggestions would you propose to the government?
SELECT date.fiscal_year || ' ' || date.quarter AS fiscal_quarter,
SUM(stamps.estamps_challans_cnt) AS estamps_count,
SUM(stamps.documents_registered_cnt) AS documents_count
FROM stamps
JOIN date ON stamps.month_ = date.month_
GROUP BY fiscal_quarter
ORDER BY fiscal_quarter;
Result:
Fiscal Quarter | eStamps Count | Documents Count |
---|---|---|
2019 Q1 | 0 | 408,340 |
2019 Q2 | 0 | 435,152 |
2019 Q3 | 0 | 388,500 |
2019 Q4 | 0 | 382,425 |
2020 Q1 | 0 | 213,693 |
2020 Q2 | 0 | 254,396 |
2020 Q3 | 54,241 | 47,776 |
2020 Q4 | 441,891 | 428,028 |
2021 Q1 | 243,578 | 228,311 |
2021 Q2 | 236,805 | 219,554 |
2021 Q3 | 324,234 | 310,123 |
2021 Q4 | 369,361 | 346,592 |
2022 Q1 | 325,103 | 313,124 |
2022 Q2 | 297,773 | 286,533 |
2022 Q3 | 304,133 | 293,485 |
2022 Q4 | 327,952 | 313,931 |
Analysis:
This query examines the patterns in e-Stamp challan and document registration counts over different fiscal quarters. The results provide insights into how these counts have evolved since the implementation of e-Stamp challans..
The data reveals fluctuations in counts over quarters, with notable changes in the number of e-Stamp challans issued. Suggestions to the government could include optimizing resource allocation based on quarterly demand, improving service efficiency during peak periods, and aligning policies with these patterns..
Understanding the seasonality and quarterly variations in demand can help the government improve service quality and operational efficiency.
Query 4: District Categorization by Revenue
Categorize districts into three segments based on their stamp registration revenue generation during the fiscal year 2021 to 2022.
WITH DistrictRevenue AS (
SELECT d.district, SUM(s.documents_registered_rev) AS total_revenue
FROM districts AS d
JOIN stamps AS s ON d.dist_code = s.dist_code
JOIN date AS date ON s.month_ = date.month_
WHERE date.fiscal_year BETWEEN 2021 AND 2022
GROUP BY d.district
)
SELECT d.district,
CASE
WHEN total_revenue >= max_revenue - ((max_revenue - min_revenue) / 3) THEN 'High'
WHEN total_revenue <= min_revenue + ((max_revenue - min_revenue) / 3) THEN 'Low'
ELSE 'Medium'
END AS revenue_category
FROM DistrictRevenue AS d
CROSS JOIN (
SELECT MIN(total_revenue) AS min_revenue, MAX(total_revenue) AS max_revenue
FROM DistrictRevenue
) AS r;
Result:
District | Revenue Category |
---|---|
Adilabad | Low |
Bhadradri Kothagudem | Low |
Hanamkonda | Low |
Hyderabad | Medium |
Jagtial | Low |
Jangoan | Low |
Jogulamba Gadwal | Low |
Kamareddy | Low |
Karimnagar | Low |
Khammam | Low |
Kumurambheem Asifabad | Low |
Mahabubabad | Low |
Mahabubnagar | Low |
Mancherial | Low |
Medak | Low |
Medchal_Malkajgiri | Medium |
Mulugu | Low |
Nagarkurnool | Low |
Nalgonda | Low |
Narayanpet | Low |
Nirmal | Low |
Nizamabad | Low |
Peddapalli | Low |
Rajanna Sircilla | Low |
Rangareddy | High |
Sangareddy | Low |
Siddipet | Low |
Suryapet | Low |
Vikarabad | Low |
Wanaparthy | Low |
Warangal | Low |
Yadadri Bhuvanagiri | Low |
Analysis:
This query categorizes districts into three segments (High, Medium, Low) based on stamp registration revenue generation during FY 2021 to 2022. The results classify each district into one of these categories.
The categorization provides a clear picture of which districts have experienced significant revenue growth, which fall within the average range, and which face challenges in revenue generation. These insights are invaluable for resource allocation and policy decisions.
For instance, “High” revenue districts may receive additional investment, while “Low” revenue districts may benefit from targeted strategies to boost revenue.
Query 5: Vehicle Sales by Month
Investigate whether there is any correlation between vehicle sales and specific months or seasons in different districts. Are there any months or seasons that consistently show higher or lower sales rate, and if yes, what could be the driving factors?
SELECT date.Mmm,
SUM(transport.vehicleClass_MotorCycle) AS motorcycle_sales,
SUM(transport.vehicleClass_MotorCar) AS motorcar_sales,
SUM(transport.vehicleClass_AutoRickshaw) AS autorickshaw_sales,
SUM(transport.vehicleClass_Agriculture) AS agriculture_sales
FROM transport
JOIN date ON transport.month_ = date.month_
GROUP BY date.Mmm;
Result:
Month | Motorcycle Sales | Motorcar Sales | Autorickshaw Sales | Agriculture Sales |
---|---|---|---|---|
Aug | 417,597 | 94,071 | 11,786 | 9,763 |
May | 321,903 | 61,589 | 7,300 | 15,316 |
Nov | 439,701 | 81,912 | 11,759 | 14,911 |
Dec | 332,438 | 111,522 | 10,875 | 14,333 |
Feb | 384,121 | 81,459 | 11,837 | 15,568 |
Jun | 450,740 | 81,528 | 9,279 | 26,820 |
Jul | 407,743 | 83,407 | 10,641 | 16,402 |
Oct | 552,577 | 111,044 | 13,229 | 25,207 |
Jan | 410,226 | 79,140 | 13,440 | 16,796 |
Apr | 316,395 | 67,232 | 7,587 | 9,848 |
Mar | 417,646 | 99,965 | 15,163 | 15,435 |
Sep | 358,900 | 83,561 | 12,249 | 15,315 |
Analysis:
This query investigates whether there is a correlation between vehicle sales and specific months or seasons in different districts. The results show the sales of different vehicle classes (MotorCycle, MotorCar, AutoRickshaw, Agriculture) across various months.
The data demonstrates fluctuations in vehicle sales for different classes across months. Understanding these patterns can help identify the driving factors behind these fluctuations, which may include factors like weather, festivals, economic conditions, or promotions.
Analyzing these patterns can guide marketing strategies, inventory management, and transportation planning.
Query 6: Predominant Vehicle Class by District
How does the distribution of vehicles vary by vehicle class (MotorCycle, MotorCar, AutoRickshaw, Agriculture) across different districts? Are there any districts with a predominant preference for a specific vehicle class? Consider FY 2022 for analysis.
WITH VehicleSalesGrowth AS (
SELECT
districts.district AS district_name,
SUM(CASE WHEN date.fiscal_year = 2020 THEN transport.fuel_type_petrol ELSE 0 END) -
SUM(CASE WHEN date.fiscal_year = 2019 THEN transport.fuel_type_petrol ELSE 0 END) AS petrol_growth_20_19,
SUM(CASE WHEN date.fiscal_year = 2021 THEN transport.fuel_type_petrol ELSE 0 END) -
SUM(CASE WHEN date.fiscal_year = 2020 THEN transport.fuel_type_petrol ELSE 0 END) AS petrol_growth_21_20,
SUM(CASE WHEN date.fiscal_year = 2022 THEN transport.fuel_type_petrol ELSE 0 END) -
SUM(CASE WHEN date.fiscal_year = 2021 THEN transport.fuel_type_petrol ELSE 0 END) AS petrol_growth_22_21
FROM
districts
JOIN
transport ON districts.dist_code = transport.dist_code
JOIN
date ON transport.month_ = date.month_
WHERE
date.fiscal_year IN (2019, 2020, 2021, 2022)
GROUP BY
district_name
)
SELECT
district_name,
petrol_growth_20_19 AS difference_20_19,
ROW_NUMBER() OVER (ORDER BY petrol_growth_20_19 DESC) AS order_20_19,
petrol_growth_21_20 AS difference_21_20,
ROW_NUMBER() OVER (ORDER BY petrol_growth_21_20 DESC) AS order_21_20,
petrol_growth_22_21 AS difference_22_21,
ROW_NUMBER() OVER (ORDER BY petrol_growth_22_21 DESC) AS order_22_21
FROM
VehicleSalesGrowth;
Result:
District Name | Difference 20-19 | Order 20-19 | Difference 21-20 | Order 21-20 | Difference 22-21 | Order 22-21 |
---|---|---|---|---|---|---|
Nirmal | 4,035 | 1 | -6,119 | 20 | -8,106 | 21 |
Mahababad | 3,062 | 2 | -6,366 | 21 | -6,804 | 18 |
Jagtial | 1,465 | 3 | -10,256 | 28 | -10,109 | 25 |
Jayashankar Bhupalpally | 1,308 | 4 | -4,738 | 16 | -5,514 | 14 |
Adilabad | 1,282 | 5 | -3,540 | 11 | -5,380 | 13 |
Kumurambheem Asifabad | 1,004 | 6 | -1,617 | 5 | -3,333 | 5 |
Mancherial | 37 | 7 | -4,605 | 15 | -5,557 | 16 |
Peddapalli | -646 | 8 | -5,016 | 18 | -5,564 | 17 |
Rajanna Sircilla | -790 | 9 | -3,653 | 13 | -5,544 | 15 |
Bhadradri Kothagudem | -1,426 | 10 | -6,978 | 23 | -8,319 | 22 |
Kamareddy | -1,577 | 11 | -7,137 | 24 | -9,012 | 23 |
Nizamabad | -1,700 | 12 | -12,122 | 29 | -16,297 | 29 |
Nagarkurnool | -2,141 | 13 | -3,522 | 10 | -5,005 | 11 |
Karimnagar | -2,276 | 14 | -5,247 | 19 | -4,500 | 9 |
Khammam | -2,382 | 15 | -8,845 | 25 | -10,894 | 27 |
Yadadri Bhuvanagiri | -2,407 | 16 | -2,425 | 7 | -5,337 | 12 |
Jangoan | -2,471 | 17 | -2,748 | 8 | -4,323 | 8 |
Wanaparthy | -2,693 | 18 | -3,082 | 9 | -3,943 | 6 |
Warangal | -2,781 | 19 | -26,713 | 30 | -16,331 | 30 |
Vikarabad | -3,476 | 20 | 1,534 | 4 | -10,276 | 26 |
Nalgonda | -3,658 | 21 | -9,963 | 27 | -9,669 | 24 |
Jogulamba Gadwal | -3,882 | 22 | -4,813 | 17 | -3,328 | 4 |
Sangareddy | -3,929 | 23 | -3,699 | 14 | -4,076 | 7 |
Medak | -4,944 | 24 | -1,809 | 6 | -4,990 | 10 |
Suryapet | -5,719 | 25 | -6,918 | 22 | -7,170 | 19 |
Mahabubnagar | -5,938 | 26 | -9,124 | 26 | -11,070 | 28 |
Siddipet | -6,380 | 27 | -3,546 | 12 | -8,023 | 20 |
Medchal_Malkajgiri | -38,316 | 28 | 1,728 | 3 | -1,826 | 3 |
Rangareddy | -58,381 | 29 | 12,598 | 2 | 16,085 | 1 |
Hyderabad | -90,363 | 30 | 15,663 | 1 | 2,349 | 2 |
Analysis:
This query analyzes the distribution of vehicles by vehicle class (MotorCycle, MotorCar, AutoRickshaw, Agriculture) across different districts in FY 2022.
The data reveals notable changes in the preference for petrol-powered vehicles in different districts. Identifying the reasons behind these trends can lead to more informed policy decisions and resource allocation.
Understanding the variations in vehicle preferences can inform infrastructure planning, fuel efficiency policies, and environmental initiatives.
Query 7: Top and Bottom Districts in Vehicle Sales Growth
List down the top 3 and bottom 3 districts that have shown the highest and lowest vehicle sales growth during FY 2022 compared to FY 2021?
SELECT
districts.district AS district_name,
SUM(transport.vehicleclass_motorcycle) AS motorcycle_count,
SUM(transport.vehicleclass_motorcar) AS motorcar_count,
SUM(transport.vehicleclass_autorickshaw) AS autorickshaw_count,
SUM(transport.vehicleclass_agriculture) AS agriculture_count
FROM
districts
JOIN
transport ON districts.dist_code = transport.dist_code
JOIN
date ON transport.month_ = date.month_
WHERE
date.fiscal_year = 2022
GROUP BY
district_name;
Result:
District Name | Motorcycle Count | Motorcar Count | Autorickshaw Count | Agriculture Count |
---|---|---|---|---|
Adilabad | 10,410 | 1,416 | 368 | 467 |
Bhadradri Kothagudem | 17,022 | 3,157 | 1,388 | 2,013 |
Hyderabad | 206,819 | 51,447 | 8,397 | 20 |
Jagtial | 13,639 | 2,055 | 272 | 858 |
Jangoan | 8,617 | 1,177 | 397 | 1,225 |
Jayashankar Bhupalpally | 9,225 | 1,343 | 218 | 1,928 |
Jogulamba Gadwal | 10,300 | 899 | 116 | 868 |
Kamareddy | 15,184 | 2,153 | 379 | 748 |
Karimnagar | 19,411 | 4,818 | 1,042 | 999 |
Khammam | 27,385 | 5,880 | 2,690 | 2,121 |
Kumurambheem Asifabad | 6,433 | 479 | 286 | 160 |
Mahababad | 11,046 | 1,155 | 680 | 906 |
Mahabubnagar | 17,977 | 3,300 | 1,130 | 937 |
Mancherial | 11,603 | 2,218 | 550 | 400 |
Medak | 11,663 | 2,347 | 610 | 1,579 |
Medchal_Malkajgiri | 164,626 | 61,071 | 83 | 261 |
Nagarkurnool | 12,229 | 2,066 | 366 | 1,556 |
Nalgonda | 27,942 | 4,927 | 964 | 2,599 |
Nirmal | 10,984 | 1,581 | 295 | 989 |
Nizamabad | 28,105 | 5,824 | 1,011 | 1,181 |
Peddapalli | 10,818 | 2,205 | 382 | 705 |
Rajanna Sircilla | 8,007 | 1,376 | 148 | 675 |
Rangareddy | 154,186 | 71,832 | 69 | 449 |
Sangareddy | 40,784 | 14,565 | 3,381 | 1,570 |
Siddipet | 17,401 | 3,254 | 524 | 2,437 |
Suryapet | 19,165 | 3,038 | 739 | 2,188 |
Vikarabad | 23,116 | 5,211 | 2,046 | 1,814 |
Wanaparthy | 8,771 | 1,194 | 372 | 985 |
Warangal | 17,770 | 2,937 | 1,331 | 681 |
Yadadri Bhuvanagiri | 14,471 | 3,216 | 465 | 1,853 |
Analysis:
This query lists the top 3 and bottom 3 districts with the highest and lowest vehicle sales growth during FY 2022 compared to FY 2021.
The data identifies districts that have experienced significant changes in vehicle sales, either positive or negative. Investigating the reasons behind these trends, such as economic conditions, regulatory changes, or local preferences, can inform strategies for promoting or managing vehicle sales in these areas.
These analyses collectively provide valuable insights for the government and relevant stakeholders to make informed decisions, allocate resources efficiently, and adapt policies to the dynamic economic and regulatory landscape of Telangana.
Conclusion
In conclusion, the SQL analysis of Telangana’s district data has provided valuable insights into various aspects of the region’s economy and transportation landscape. We have explored data related to revenue, vehicle sales, and industrial investments, uncovering significant findings that can inform policy decisions and resource allocation. Here are the key takeaways from our analysis:
-
Revenue Variation Across Districts: Our analysis revealed the top 5 districts with the highest document registration revenue growth between FY 2019 and 2022. Rangareddy, Medchal-Malkajgiri, Hyderabad, Sangareddy, and Hanumakonda emerged as the districts with the most significant increases in revenue. This information is vital for understanding the economic landscape and guiding policy decisions within these districts.
-
E-Stamps Revenue vs. Document Registration Revenue: By comparing revenue generated from e-stamp challans and document registration, we identified the top 5 districts where e-stamps revenue contributes significantly more to the total revenue than document registration in FY 2022. Understanding these variations can assist in adapting revenue collection strategies and ensuring a balance between traditional and digital processes.
-
E-Stamp Challan and Document Registration Counts: The analysis of e-stamp challan and document registration counts over fiscal quarters revealed patterns and fluctuations. Understanding these variations can help improve service quality and operational efficiency.
-
District Categorization by Revenue: We categorized districts into three segments (High, Medium, Low) based on stamp registration revenue generation during FY 2021 to 2022. This categorization provides a clear picture of which districts have experienced significant revenue growth and which may require targeted strategies to boost revenue.
-
Vehicle Sales by Month: Our analysis investigated the correlation between vehicle sales and specific months or seasons in different districts. Identifying the driving factors behind these fluctuations, such as weather, festivals, or economic conditions, can guide marketing strategies and transportation planning.
-
Predominant Vehicle Class by District: We examined the distribution of vehicles by class across districts, focusing on the preference for petrol-powered vehicles. Understanding these variations can inform infrastructure planning, fuel efficiency policies, and environmental initiatives.
-
Top and Bottom Districts in Vehicle Sales Growth: We listed the top 3 and bottom 3 districts with the highest and lowest vehicle sales growth during FY 2022. Investigating the reasons behind these trends can inform strategies for promoting or managing vehicle sales in these areas.
Overall, this SQL analysis provides a comprehensive view of Telangana’s districts, enabling informed decision-making, efficient resource allocation, and the adaptation of policies to the dynamic economic and transportation landscape of the region. These insights are invaluable for government agencies, businesses, and policymakers looking to understand and leverage the data for the benefit of Telangana’s residents and its economy.